During last night's GTC keynote, Nvidia CEO announced a series of blockbuster chip products, including not only the H800 chip, which is specially prepared for China and is based on the H100 revision. At the same time, the company also announced products prepared for generative AI.
In this keynote, Huang Renxun also brought a series of products such as accelerated 2nm design computational lithography, which we now summarize and share with our readers.
Adjust the flagship H100 to H800 for export to China
According to Reuters, the American semiconductor design company Nvidia, which leads the artificial intelligence chip market, has modified its flagship product to a version that can be legally exported to China.
Last year, US regulatory agencies established rules prohibiting Nvidia from selling its two most advanced chips, the A100 and the newer H100, to Chinese customers. These chips are crucial for developing generative artificial intelligence technology, such as OpenAI's ChatGPT and similar products.
Advertisement
In November, Reuters reported that Nvidia designed a chip called the A800, which reduced some functions of the A100, making the A800 legally exportable to China.
On Tuesday, the company confirmed that it has developed a similar version of the H100 chip for export to China. Alibaba Group Holding, Baidu Inc., and Tencent Holdings, and other Chinese technology companies' cloud computing departments are using this new chip called H800, said an Nvidia spokesperson.
US regulatory agencies implemented rules last fall to slow down China's development in key technology areas such as semiconductors and artificial intelligence.
The rules surrounding artificial intelligence chips imposed a test that prohibits chips with strong computing power and high chip-to-chip data transfer rates. The transfer rate is very important when training artificial intelligence models with a large amount of data, because slower transfer rates mean more training time.
A Chinese chip industry source told Reuters that the H800 mainly reduces the chip-to-chip data transfer rate to about half of the flagship H100 rate.Nvidia spokesperson refused to disclose the differences between the H800 and H100 for China, merely stating, "Our 800 series products fully comply with export control regulations."
Breaking through computational lithography, laying the foundation for 2nm chip manufacturing
At Nvidia's GTC conference this time, the breakthrough in computational lithography by ASML, TSMC, and Synopsys to help the industry surpass physical limitations is another highlight worth paying attention to.
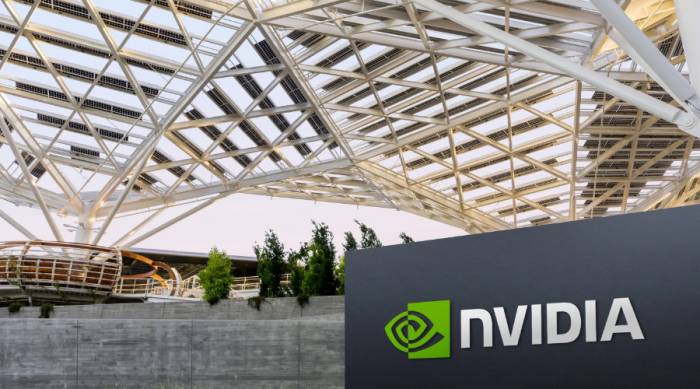
NVIDIA stated that it will bring accelerated computing into the field of computational lithography, enabling semiconductor leaders such as ASML, TSMC, and Synopsys to accelerate the design and manufacturing of the next generation of chips, just as the current production process is approaching the limits of physics to make it possible.
In a press release, Nvidia pointed out that the new NVIDIA cuLitho software library for computational lithography has been integrated by the world's leading wafer foundry TSMC and the leader in electronic design automation, Synopsys, into its latest generation of NVIDIA Hopper™ architecture GPU software, manufacturing processes, and systems. Equipment manufacturer ASML has worked closely with NVIDIA in terms of GPUs and cuLitho, and plans to integrate support for GPUs into all of its computational lithography software products.
This advancement will enable chips to have finer transistors and wires than currently available, while accelerating time to market and improving the energy efficiency of large data centers that operate 24/7 to drive the manufacturing process.
"The chip industry is the foundation of almost all other industries in the world," said NVIDIA founder and CEO Huang Renxun. "As lithography technology reaches its physical limits, NVIDIA launches cuLitho and cooperates with our partners TSMC, ASML, and Synopsys, enabling wafer factories to increase output, reduce carbon footprint, and lay the foundation for 2nm and higher processes."
Running on GPUs, cuLitho provides up to 40 times the performance leap over current lithography technology (the process of creating patterns on silicon wafers), accelerating a large amount of computational workloads that currently consume tens of billions of CPU hours each year.
It enables 500 NVIDIA DGX H100 systems to complete the work of 40,000 CPU systems, running all parts of the computational lithography process in parallel, helping to reduce power demand and potential environmental impact.
In the short term, wafer factories using cuLitho can help produce 3-5 times more photomasks per day—the templates for chip design—using nine times less power than the current configuration. Photomasks that take two weeks to complete can now be completed overnight.In the long term, cuLitho will enable better design rules, higher density, higher yield, and AI-driven lithography.
"The cuLitho team has made commendable progress in accelerating computational lithography by shifting expensive operations to GPUs," said Dr. CC Wei, CEO of TSMC. "This development opens up new possibilities for TSMC to deploy inverse lithography technology and lithographic solutions such as deep learning more broadly in chip manufacturing, making an important contribution to the continued scaling of semiconductors."
"We plan to integrate support for GPUs into all of our computational lithography software products," said Peter Wennink, CEO of ASML. "Our collaboration with NVIDIA on GPUs and cuLitho should bring significant benefits to computational lithography, thereby benefiting semiconductor scaling. This is especially true in the era of High NA extreme ultraviolet lithography."
Aart de Geus, Chairman and CEO of Synopsys, said: "Computational lithography, especially optical proximity correction (OPC), is pushing the computational workload limits of the most advanced chips." By collaborating with our partner NVIDIA to run Synopsys OPC software on the cuLitho platform, we have increased performance from weeks to days! The collaboration between our two leading companies will continue to drive amazing progress in the industry."
NVIDIA stated that in recent years, the computational time cost required for the largest workload in semiconductor manufacturing has exceeded Moore's Law due to the increased number of transistors in newer nodes and stricter precision requirements. Future nodes will require more detailed calculations, and not all of these can be accommodated by the available computing bandwidth provided by current platforms, slowing the pace of semiconductor innovation.
Wafer fab process changes often require OPC revisions, causing bottlenecks. cuLitho helps eliminate these bottlenecks and makes new solutions and innovative technologies possible, such as curved masks, High NA EUV lithography, and sub-atomic photoresist modeling required for new technology nodes.
Nvidia Announces BlueField-3 GA
Nvidia today announced the full launch of its BlueField-3 data processing unit (DPU) and impressive early deployments, including Oracle Cloud Infrastructure. First described in 2021, BlueField-3 is now delivered and is Nvidia's third-generation DPU, with approximately 22 billion transistors. Compared to the previous generation BlueField, the new DPU supports Ethernet and InfiniBand connections with speeds up to 400 gigabits per second, computational performance increased by 4 times, encryption acceleration increased by 4 times, storage processing speed increased by 2 times, and memory bandwidth increased by 4 times."
Nvidia CEO Huang Renxun said in the GTC 23 keynote speech: "In modern software-defined data centers, the operating systems that perform virtualization, networking, storage, and security consume nearly half of the CPU cores and related power in the data center. Data centers must accelerate each workload to reclaim power and release CPUs for revenue-generating workloads. Nvidia BlueField offloads and accelerates data center operating systems and infrastructure software."
As early as 2020, Nvidia formulated a DPU strategy, believing that CPUs are mired in internal chores such as those cited by Huang. Nvidia believes that DPU will absorb these tasks, thereby releasing CPUs for applications. Other chip suppliers, especially Intel and AMD, seem to agree and have jumped into the DPU market.Sometimes described as smart network cards of the steroid class, interest in DPU (Data Processing Unit) has been piqued, but it has not yet translated into widespread sales. Change may now be on the horizon. Huang cited "more than 20 ecosystem partners," including Cisco, DDN, Dell EMC, and Juniper, which are now using BlueField technology.
At a media/analyst pre-briefing, NVIDIA Networking Vice President Kevin Deierling stated: "BlueField-3 is fully in production and available for use. It has twice the number of Arm processor cores as BlueField-2, more accelerators, and runs workloads eight times faster than our previous generation DPU. BlueField-3 can offload, accelerate, and isolate workloads across cloud HPC, enterprise, and accelerated AI use cases."
NVIDIA's DPU is aimed at supercomputers, data centers, and cloud providers. At GTC, NVIDIA touted the Oracle Cloud deployment, where BlueField-3 is part of NVIDIA's larger DGX-in-the-Cloud victory.
"As you have heard, we announced that Oracle Cloud Infrastructure is the first to run DGX Cloud and AI supercomputing services, enabling enterprises to immediately access the infrastructure and software needed for generating advanced AI training models. OCI [also] selected BlueField-3 to achieve higher performance, efficiency, and security. Compared to BluField-2, BlueField-3 provides a significant performance and efficiency boost by offloading data center infrastructure tasks from the CPU, increasing virtualization instances by eight times," Deierling said.
In the official announcement, NVIDIA quoted Oracle Cloud Infrastructure Executive Vice President Clay Magouyrk as saying: "Oracle Cloud Infrastructure provides enterprise customers with almost unparalleled accessibility to artificial intelligence and scientific computing infrastructure, with the potential to change the industry. The NVIDIA BlueField-3 DPU is a key part of our strategy to provide the most advanced, sustainable cloud infrastructure and ultimate performance."
Other victories for BlueField-3 in CSP include Baidu, CoreWeave, JD.com, Microsoft Azure, and Tencent.
NVIDIA also reported that BlueField-3 has "full backward compatibility through the DOCA software framework."
DOCA is the programming tool for BlueField, and DOCA 2.0 is the latest version. NVIDIA has been steadily adding features to its DPU product line. For example, recently, it enhanced inline GPU packet processing "to implement high data rate solutions: data filtering, data placement, network analysis, sensor signal processing, etc." The new DOCA GPUNetIO library can overcome some limitations previously found in DPDK solutions.According to Nvidia, Nvidia's real-time GPU packet processing is a technology useful for a variety of different application fields, including signal processing, network security, information gathering, and input reconstruction. The goal of these applications is to achieve an inline packet processing pipeline to receive packets in GPU memory (without having to temporarily store a copy through CPU memory); process them in parallel with one or more CUDA cores; then run inference, evaluation, or send the results of the computation over the network.
Introducing the H100 NVL, a memory server card for large models
Anandtech states that although this year's spring GTC event did not feature any new GPUs or GPU architectures from NVIDIA, the company is still launching new products based on last year's Hopper and Ada Lovelace GPUs. However, in the high-end market, the company announced today a new variant of the H100 accelerator specifically for large language model users: the H100 NVL.
The H100 NVL is an interesting variant of the NVIDIA H100 PCIe card, a symbol of the times and NVIDIA's extensive success in the field of AI, targeting a single market: the deployment of large language models (LLM). There is something that makes this card different from NVIDIA's usual server fare—most notably, its two H100 PCIe boards have been bridged together—but the biggest gain is the large memory capacity. The combined dual-GPU card provides 188GB of HBM3 memory—94GB per card—offering more memory per GPU than any other NVIDIA part to date, even within the H100 series.
Driving this SKU is a specific niche market: memory capacity. Large language models like the GPT series are limited in many ways by memory capacity, as they can quickly fill up the H100 accelerator to save all their parameters (175B in the case of the largest GPT-3 model). Therefore, NVIDIA chose to piece together a new H100 SKU that provides a little more memory per GPU than their usual H100 parts, which top out at 80GB per GPU.
Under the hood of the package, what we see is essentially a special container for the GH100 GPU placed on the PCIe card. All GH100 GPUs come with six HBM memory stacks (HBM2e or HBM3), each with a capacity of 16GB. However, for yield reasons, NVIDIA only offers five of the six HBM stacks in its regular H100 parts. So, although each GPU is nominally equipped with 96GB of VRAM, only 80GB is available on the regular SKU.
The H100 NVL is the mythical fully enabled SKU, enabling all six stacks. By turning on the sixth HBM stack, NVIDIA was able to access the additional memory and additional memory bandwidth it provides. It will have some substantial impact on yield—how much is a closely guarded secret of NVIDIA—but the LLM market is obviously large enough and willing to pay a high enough premium for a nearly perfect GH100 package to make it worth NVIDIA's while.
Even so, it should be noted that customers cannot access the full 96GB per card. Instead, in the total memory capacity of 188GB, their effective capacity per card is 94GB. Before today's keynote, NVIDIA did not elaborate on this design in our pre-briefing, but we suspect that this is also due to yield reasons, allowing NVIDIA some leeway in disabling bad units (or layers) in the HBM3 memory stack. The end result is that the new SKU provides 14GB of memory for each GH100 GPU, an increase of 17.5% in memory. At the same time, the total memory bandwidth of the card is 7.8TB/s, and the total memory bandwidth of a single board is 3.9TB/s.
In addition to the increased memory capacity, the individual cards in the larger dual-GPU/dual-card H100 NVL look a lot like the H100 SXM5 version placed on the PCIe card in many ways. Although the regular H100 PCIe is somewhat limited by the use of slower HBM2e memory, fewer active SM/tensor cores, and lower clock speeds, the tensor core performance data that NVIDIA quotes for the H100 NVL is exactly the same as the H100 SXM5, indicating that the card is not further reduced like the regular PCIe card. We are still waiting for the final, complete specifications of the product, but assuming that everything here is as presented, then the GH100 entering the H100 NVL will represent the highest available tier of the GH100 currently available.
The plural needs to be emphasized here. As mentioned earlier, the H100 NVL is not a single GPU part, but a dual-GPU/dual-card part, which is presented to the host system in this way. The hardware itself is based on two H100s in the PCIe form factor, which are bridged together with three NVLink 4s. Physically, this is actually exactly the same as NVIDIA's existing H100 PCIe design—the latter can already be paired with NVLink bridges—so the difference is not in the structure of the two-board/four-slot behemoth, but in the quality of the internal chips. In other words, you can bundle ordinary H100 PCIe cards together today, but they cannot match the memory bandwidth, memory capacity, or tensor throughput of the H100 NVL.Surprisingly, despite the impressive specifications, the TDP has remained almost unchanged. The H100 NVL is a 700W to 800W component, broken down into 350W to 400W per board, with its lower limit matching the TDP of a regular H100 PCIe. In this case, NVIDIA seems to prioritize compatibility over peak performance, as few server chassis can handle more than 350W for a PCIe card (even fewer over 400W), which means the TDP needs to stay stable. However, it is unclear how NVIDIA can provide additional performance, considering the higher performance data and memory bandwidth. Power binning can play a significant role here, but it may also be the case that NVIDIA provides the card with higher boost clock speeds than usual, as the target market primarily focuses on tensor performance and will not light up the entire GPU at once.
Otherwise, given NVIDIA's general preference for SXM components, NVIDIA's decision to release what is essentially the best H100 bin is an unusual choice, but it makes sense in the context of the demands of LLM customers. Large H100 clusters based on SXM can easily scale up to 8 GPUs, but the amount of NVLink bandwidth available between any two GPUs is limited by the need to go through the NVSwitch. For a configuration with only two GPUs, pairing a set of PCIe cards is much more straightforward, with a fixed link guaranteeing a bandwidth of 600GB/s between the cards.
But perhaps more importantly than this is the ability to quickly deploy the H100 NVL within existing infrastructure. LLM customers do not need to install an H100 HGX carrier board specifically built for paired GPUs; they can simply add the H100 NVL to a new server build or as a relatively quick upgrade to an existing server build. After all, NVIDIA is targeting a very specific market here, so the usual advantages of SXM (and NVIDIA's ability to leverage its collective influence) may not apply.
In summary, according to NVIDIA, the H100 NVL offers a GPT3-175B inference throughput 12 times that of the previous generation HGX A100 (8 H100 NVLs vs. 8 A100s). This is certainly attractive for customers who want to deploy and scale systems for LLM workloads as quickly as possible. As mentioned earlier, the H100 NVL does not bring any new architectural features—most of the performance gains here come from the new transformer engines of the Hopper architecture—but the H100 NVL will serve as the fastest PCIe H100 option for specific niche markets, as well as the option with the largest GPU memory pool.
In conclusion, according to NVIDIA, H100 NVL cards will begin shipping in the second half of this year. The company has not quoted prices, but for a product that is essentially the top GH100, we expect them to command the highest prices. Especially considering how the surge in LLM usage has turned into a new gold rush for the server GPU market.
Nvidia's "Cloud", Services Starting at $37,000
If you are a loyal supporter of Nvidia, be prepared to spend a lot of money to use its AI factory in the cloud.
Nvidia co-founder and CEO Huang Renxun mentioned the plan for Nvidia DGX Cloud last month when discussing the GPU manufacturer's quarterly earnings, essentially calling for the company's DGX AI supercomputer hardware and accompanying software—especially its extensive enterprise AI suite of software—to be put on a public cloud platform for enterprise use.
We must declare that Nvidia is not rich enough, or foolish enough, to build a cloud to compete with companies like Amazon Web Services, Microsoft Azure, or Google Cloud. But they are smart enough to take advantage of these massive computing and storage utilities for their own profit, and to make money by selling services on top of the infrastructure they have built, which is based on their own components.
The cleverness of DGX Cloud is not in having a certified local and cloud stack to run Nvidia's AI hardware and software. You need to pay Nvidia to do this in a SaaS model—Nvidia can sell you the components to build the infrastructure.In and of itself, this is the latest attempt to democratize AI, taking it out of the realm of HPC and research institutions and placing it within the reach of mainstream businesses eager to leverage the business advantages that emerging technologies can deliver.
For Nvidia, the AI-as-a-Service of the DGX Cloud represents a strong shift towards a cloud-first strategy, as well as an understanding—like other component manufacturers—that it is now both a hardware manufacturer and a software company, with the public cloud being a natural way to make that software easily accessible and, more importantly, to monetize it.
This is an important next step for a company that placed AI at the center of its forward strategy more than a decade ago, building a roadmap centered around AI. Nvidia launched the DGX-1, its first deep learning supercomputer, in 2016. The fourth-generation system was introduced last year. The first DGX SuperPODs emerged in 2020, and a year later, Nvidia launched AI Enterprise, a software suite that includes frameworks, tools, and a substantial dose of VMware vSphere.
AI Enterprise highlights the increasing importance of software to Nvidia—reflecting a similar trend among other component manufacturers—this company now employs more people in software than in hardware.
With the DGX Cloud, Nvidia can now deliver all of this to businesses that wish to leverage generative AI tools in their workflows, such as the widely popular ChatGPT from OpenAI (via Microsoft), but do not have the resources to expand their internal infrastructure data centers to support it. They can now access it via the cloud, enjoying all its scalability and pay-as-you-go benefits.
Manuvir Das, Nvidia's Vice President of Enterprise Computing, told reporters at the pre-GTC conference: "For years, we have been working with enterprise companies to create their own models to train their own data." "In the past few months, services based on very large GPT models like ChatGPT have become increasingly popular, with millions of people using a single model every day. When we work with enterprise companies, many of them are interested in creating models for their own purposes using their own data."
According to the latest introduction, the DGX Cloud, which rents Nvidia's comprehensive cloud AI supercomputer, starts at $36,999 per instance per month. The rental includes the use of a cloud computer with eight Nvidia H100 or A100 GPUs and 640GB of GPU memory. The price includes AI Enterprise software for developing AI applications and large language models, such as BioNeMo.
"DGX Cloud has its own pricing model, so customers pay Nvidia, and they can purchase it through any cloud marketplace based on the location they choose to use it, but this is a service priced by Nvidia, including all fees," said Nvidia's Vice President of Enterprise Computing, Manuvir Das, at a press conference.
The starting price of DGX Cloud is nearly twice that of the $20,000 per month that Microsoft Azure charges for a fully loaded A100 instance, which has 96 CPU cores, 900GB of storage, and 8 A100 GPUs per month.
Oracle hosts the DGX Cloud infrastructure in its RDMA supercluster, which can scale up to 32,000 GPUs. Microsoft will launch DGX Cloud next quarter, followed by Google Cloud.Customers will have to pay an additional fee for the latest hardware, but the integration of the software library and tools may attract businesses and data scientists.
Nvidia claims it offers the best available hardware for AI. Its GPUs are the cornerstone of high-performance and scientific computing. However, Nvidia's proprietary hardware and software are like using an Apple iPhone—once you get the best hardware, it's hard to get out of the lock-in, and it can cost a lot of money throughout its lifecycle.
But paying a premium for Nvidia's GPUs may bring long-term benefits. For example, Microsoft is investing in Nvidia's hardware and software because it offers cost savings and greater revenue opportunities through Bing with AI.
The concept of the AI factory was proposed by CEO Huang Renxun, who envisions data as raw materials and the factory as a place to transform it into usable data or sophisticated AI models. Nvidia's hardware and software are the main components of the AI factory.
"You just provide your work, point to your datasets, and click start, and all the orchestration and everything below is handled in the DGX Cloud," said Nvidia's Vice President of Enterprise Computing, Manuvir Das, at a press conference.
Das said that millions of people are using ChatGPT-style models, which require high-end AI hardware.
The DGX Cloud further promotes Nvidia's goal of selling its hardware and software as a suite of products. Nvidia is entering the software subscription business, which has a long tail of selling more hardware, thereby generating more software revenue. The Base Command Platform software interface will allow companies to manage and monitor DGX cloud training workloads.
Oracle Cloud has clusters with up to 512 Nvidia GPUs, as well as an RDMA network with a throughput of 200 GB per second. The infrastructure supports multiple file systems, including Lustre, with a throughput of 2 TB per second.
Nvidia also announced that more companies have adopted its H100 GPU. Amazon announced that their EC2 "UltraClusters" and P5 instances will be based on the H100. "These instances can be scaled up to 20,000 GPUs using their EFA technology," said Nvidia's Vice President of Hyperscale and HPC Computing, Ian Buck, at a press conference.
EFA technology refers to the Elastic Fabric Adapter, which is a network implementation orchestrated by Nitro, a universal custom chip that handles networking, security, and data processing.Meta Platforms has begun deploying H100 systems in Grand Teton, which is the platform for the social media company's next-generation AI supercomputer.
Summary
At the GTC that opened yesterday, Nvidia also brought a variety of products, such as the Nvidia L4 GPU for specific inference. It is reported that this GPU can provide 120 times the artificial intelligence video performance of the CPU. It offers enhanced video decoding and transcoding capabilities, video streaming, augmented reality, and generative AI video.
In addition, Nvidia has also partnered with customers to create the generative AI supercomputer Tokyo-1, which consists of 16 DGX H100 systems, each equipped with eight H100 GPUs. According to Nvidia's AI trigger mathematical calculations, this is equivalent to about half of the exaflop AI capabilities; since each H100 (which will initially have 128) provides 30 teraflops of peak FP64 power, it should reach a peak of about 3.84 petaflops.
It can be seen that Huang Renxun is leading Nvidia into a new phase.
Post Comment